In the world of product development, understanding the needs and wants of users is paramount to creating successful products.
In this episode, Hannah Clark is joined by Craig Watson—Founder & CEO of Arro—to share the secrets to successful product discovery and how you can leverage generative AI in the user research process.
Interview Highlights
- Craig’s Journey: From Startups to Arro [01:02]
- Craig has 15 years of experience in product roles, beginning in startups in Ireland.
- He worked on a music tech startup called Soundwave, which was VC backed and got acquired by Spotify in 2016.
- Craig spent five years at Spotify, experiencing significant growth from less than a thousand to over 3,000 employees in R&D.
- He worked at Spotify’s Stockholm office on the growth team and then moved to London to help with product initiatives like TEO and Spotify Duo.
- Craig transitioned into consulting, focusing on product discovery and helping companies improve their research practices.
- About a year and a half ago, Craig and his co-founder Johannes saw an opportunity to build interesting tech related to foundation Large Language Models (LLMs), leading to the founding of Arro.
- The Art and Science of Product Discovery [03:14]
- Craig finds product discovery fascinating due to its blend of science and art.
- He enjoys understanding customer needs and requirements, considering it an impactful but often overlooked aspect of product management.
- Craig appreciates the earlier part of the product management funnel where the focus is on understanding what product to build.
- He sees the art in knowing what questions to ask and being aware of potential biases in the discovery process.
- Learning from Mistakes in User Research [04:15]
- Craig acknowledges making classic mistakes in user research, including jumping straight into solution mode and validation exercises.
- He highlights the common pitfall among product managers, emphasizing the need for a different mindset that focuses on falsification rather than validation.
- He shares a personal experience from his twenties while working on Soundwave, where they focused more on evaluative rather than generative research.
- Craig emphasizes the importance of putting ego aside, listening to users, and understanding where improvements can be made in workflows.
Put your ego aside and listen to people to understand where the gaps lie and how you can improve their workflows, and build your product around that.
Craig Watson
- The Role of AI in Product Discovery [06:51]
- Craig outlines a process of starting with understanding customer jobs and problems as the foundation of the discovery process.
- He breaks down the steps involved in the research process, including formulating study objectives, recruiting participants, selecting methodologies, synthesizing information, sharing insights internally, and taking action.
- Within each job, there are various outcomes and considerations, such as data compliance, recruitment methods, and engagement strategies.
- He emphasizes the importance of focusing on workflow-based approaches, particularly in B2B contexts, rather than generic labels or categories.
- Craig discusses the challenges with traditional customer interview processes, highlighting the significant time and effort required for tasks like scheduling, transcribing, synthesizing, and analyzing interviews.
- He identifies a gap in the process where AI could potentially augment or replace certain tasks related to conducting moderated interviews.
- Arro’s product strategy is built around addressing this gap in the research process using AI technology.
- The Future of AI in Product Management [10:14]
- Craig discusses the potential for disruption by LLMs in product research, particularly in turning structured data into meaningful insights.
- Arro’s focus on generative AI aims to leverage LLMs for conducting interviews and synthesizing insights at scale.
- While AI adoption in tech is prevalent, Craig notes a slower uptake in the workplace due to various factors like security concerns and uncertainty about use cases.
- Content generation emerges as a common use case for AI, including writing user stories, research proposals, and discussion guides.
- Additionally, AI aids in content analysis, with tools like Grain enabling transcript-based querying to extract valuable insights from conversations.
- Despite early challenges, Craig finds the evolving landscape of AI adoption in product discovery promising and exciting.
- Finding Product-Market Fit with AI [15:49]
- Craig reflects on PMF as a never-ending journey and a compound play with various approaches.
- Craig shares insights from his time at Spotify, emphasizing the time and effort required to change the growth curve and achieve PMF.
- He discusses the necessity of spending time making bets, doing experiments, and making the right decisions to achieve PMF.
- Craig sees AI as a tool to reduce the time required for tasks related to finding PMF, acknowledging that while it won’t provide a perfect solution quickly, it can expedite progress towards achieving PMF.
- Caveats and Considerations for AI Integration [18:18]
- Craig acknowledges the potential negative impact of poorly executed AI features, emphasizing the need for compliance and ethical considerations.
- He highlights the tendency for companies to force AI integration without fully understanding customer needs or job requirements.
- He warns against falling into the trap of “shiny object syndrome,” where AI features are added merely for their novelty rather than practical value.
- Craig advocates for focusing on first principles and understanding users’ needs to determine whether AI integration will improve efficiency, reduce costs, or enhance the user experience.
- He emphasizes the importance of investing time and resources in AI features that directly contribute to solving users’ problems and enhancing the core functionality of the product.
- Advice for Founders and Product Managers [21:21]
- Craig emphasizes the importance of considering the context and stage of development of the startup.
- He highlights the different approaches based on the startup’s stage: finding the right market fit or addressing a known problem.
- Craig warns against the approach of building a solution and then looking for a problem it fits, acknowledging that it happens but isn’t ideal.
- Craig suggests that relationship building and generative research are crucial for early-stage startups to understand customer pain points.
Building relationships is crucial. Engaging with potential customers, understanding their pain points, and conducting generative research aligned with your value proposition is where you need to be.
Craig Watson
Meet Our Guest
Craig Watson is the founder and CEO of Arro, an AI-powered user research tool. Craig’s passion for product discovery throughout his career has given him some great insights into ways the user research process can be simplified for scale-ups and other product teams.
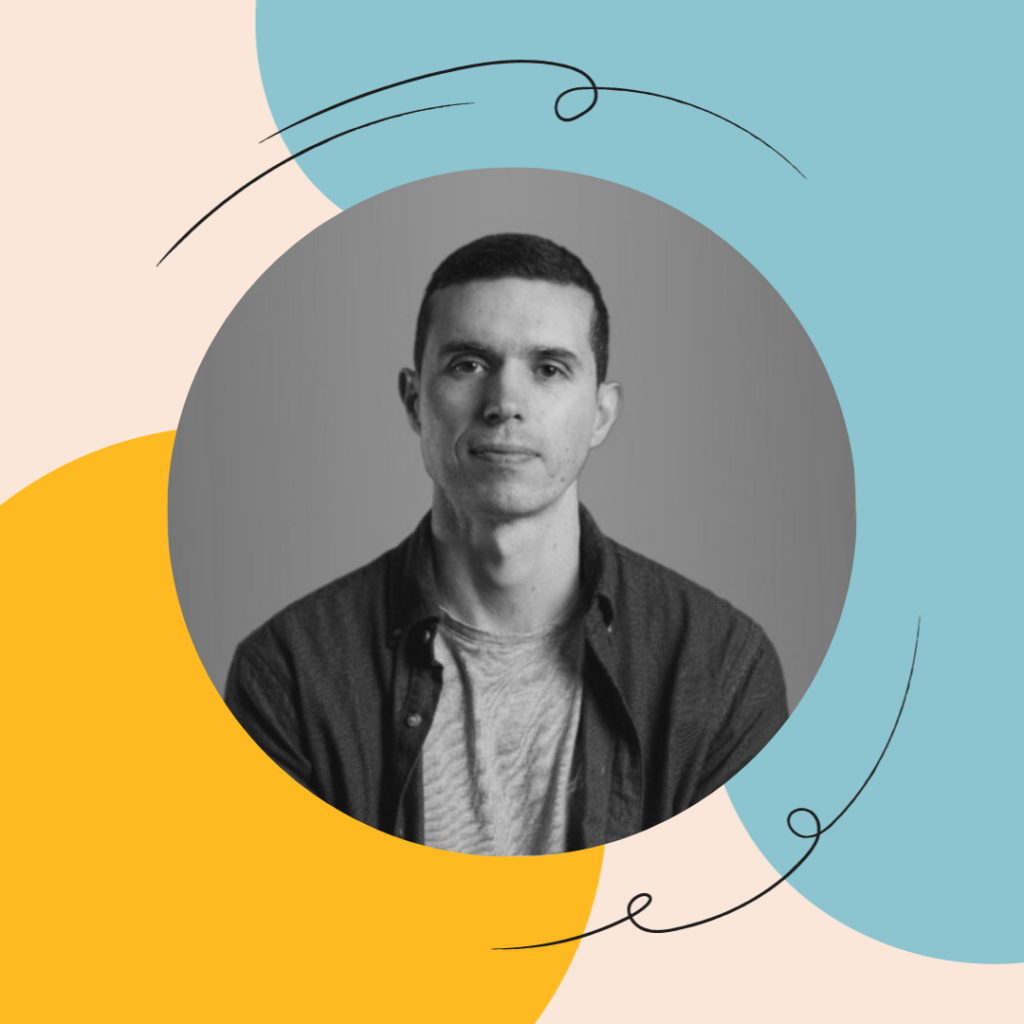
What has always drawn me into product discovery is the mix of science and art. It’s intriguing to delve into understanding customers’ wants, requirements, and needs. However, there’s also an art form to it—knowing what questions to ask and recognizing potential biases.
Craig Watson
Resources from this episode:
- Subscribe to The Product Manager newsletter
- Connect with Craig on LinkedIn
- Check out Arro
Related articles and podcasts:
- About The Product Manager Podcast
- How To Find Product-Market Fit Quickly And Cost Effectively
- How To Create An Effective Customer Feedback Loop For Product Teams
- What Does A Product Manager Do? 7 Day To Day Roles & Tasks
- The Product Development Process: How To Bring Great Ideas To Market
- Product Engagement: Are Users Loving or Leaving Your Product?
Read The Transcript:
We’re trying out transcribing our podcasts using a software program. Please forgive any typos as the bot isn’t correct 100% of the time.
Hannah Clark: I know I'm preaching to the choir, but I'm going to say it anyway. Products, above all, need to be useful. But there's still no shortage of products that were built based on a pile of assumptions about what users actually want. So why are so many orgs neglecting the product discovery process? Well, I'm sure any product manager who has also acted as the de-facto user research lead can tell you why. It's time consuming, it's expensive, and it's really, really hard to not only get a great sample size of customer data, but also to synthesize that data into concrete action.
My guest today is Craig Watson, founder and CEO of Arro, an AI-powered user research tool. Craig's passion for product discovery throughout his career has given him some great insights into ways the user research process can be simplified for scale-ups and other product teams. And you'll hear him share some great actionable tactics for doing product discovery better with generative AI. Let's jump in.
Welcome back to the Product Manager Podcast. I'm here with Craig Watson. He's the founder and CEO of Arro.
Craig, thank you so much for joining us today.
Craig Watson: Thanks a lot. I'm excited to be here.
Hannah Clark: So Craig, can you tell us a little bit about your professional background and how you ended up where you are today at Arro?
Craig Watson: Sure. Yeah, so I've been working in product roles for basically 15 years and I started off in more of a startup capacity. So we had a few startups based out of Ireland where we were building straight out of college, trying to learn as we are taking on these projects and really getting to grips with how you actually build products for consumers at the time.
And after about four years, we moved on to, there was a music tech company we started in 2012 called Soundwave. And so that was a classic kind of VC backed startup. Again, lots of highs and lows, learned very quickly on the job and probably learned more about product discovery at that point, which we'll get into on this discussion.
But what I really loved about that experience was it was so early in our careers, we were forced to do so many different roles as a product manager, you realize that you have to do so many different types of activities, right? From user research to delivery to product management at different times.
So we ended up getting acquired by Spotify in 2016 and moved in there, which was a really good experience. I wasn't planning on staying that long, but I ended up staying for five years. So that was a great experience, what Spotify was growing very quickly at the time. I think there was probably less than a thousand people in R&D when I joined, I think it was close to like 3,000 plus by the time I left, you know, it's a huge growth.
So I worked at the Stockholm office for a couple of years on the growth team. And then I moved over to London when they set up an office going to help spin up some of the product initiatives for TEO, select the kids app and Spotify Duo, a few other pieces. So that was very different, obviously from the startup world, very different environments to be a product manager, but I really enjoyed it.
And then most recently, I actually went into consulting for about a year and a half where I was working specifically on product discovery and trying to help other companies improve their research practices and learn how to get in front of customers, make evidence backed decisions, and basically help them build better products.
Just to kind of take it off. So what happened then was realized about a year and a half ago, there was an opportunity to build some interesting tech, the way things are evolving with foundation LLMs. So myself and my co-founder Johannes got together. I could tell you a bit more about that in due course.
Hannah Clark: Absolutely. So before we jumped in, can you tell us a little bit about product discovery in general and why it's become this passion of yours?
Craig Watson: So I think what's always drawn me into product discovery has been, I guess, the mix of science and art. It's interesting to think about how do you really understand what customers want, what the requirements are, what their needs are. But then there's also still an art form to it, understanding what questions to ask, knowing what's potentially leading or biased.
And I think I've always enjoyed the delivery part of product management as a craft, but it's been that earlier part of the funnel where you're actually trying to understand what product to build. That I think is just often overlooked, usually impactful, and for whatever reason, just the way my personality is, I've been drawn to it because I find it just fascinating.
Hannah Clark: Yeah, of course, nothing more fascinating than understanding what people really want. So when you think of your past work in product discovery, before AI became the tool that it is now, what's been your process for understanding user pain points more profoundly and deciding what to build?
Craig Watson: So I think it's worth also mentioning.
We made a lot of all the classic mistakes that you make when you start to do user research and we've learned the hard way where we would, sometimes jump straight into solution mode and do the classic validation exercise and try to prove something is right.
And I think that's probably the most common pitfall I've seen with product managers, specifically, it takes a very different mindset to falsify. And it's quite a hard thing to do because you have an idea and you're excited about the idea and you've got to rally people around that idea and you've got to get stakeholders interested, but effectively the good researchers and like the scientific process is falsification.
Right? It's actually trying to go out there and see what doesn't work. So it took us a while to really adapt to that kind of mentality. And I think part of that then is also the methodology when you're asking questions, making sure you're not leading, being objective, et cetera. I think also just being like, I can give you a little bit of a backstory.
I think the first time we were in our twenties, it was Soundwave was kind of taking off, we had million plus installs on the app and we were trying to build a new feature. We were doing one-on-one conversations with people. We were doing it in person actually at the time before it became more common to do remotely.
And we were learning quickly about what people wanted. We were excited about what was being built, but it was quite self referential in some ways because it was quite a small feature set that we were exploring. We weren't really getting into the problems. It wasn't generative. It was more evaluative.
It was like, here's some things we're thinking of building. We're trying to learn a bit more about their interests and music specifically at the time. And we actually brought in a third party, an executive who was helping consulting with us and he was helping do some of these interviews. And he said, look, I think what you're doing is fine, but you've got to be wary that there's going to be local maximums here.
And like you're optimizing the product, but the stage at your ass, your startup, you know, I think you really need to think about more generative research, more foundational research, understand the jobs that people want to understand the context, what are the outcomes that they're trying to drive towards.
And then start building your solution around that. And so that's like another big learning. Another hard thing to do when you're a kind of a solution mindset and you want to come out and optimize and you feel like you've got these great ideas is again, just to put your ego away and just listen to people and understand like, where are the holes, where are the steps that you could improve their workflows and really build your product around that.
So, don't think I have it nailed and obviously definitely need some help. I've worked with some amazing researchers over the years and data scientists. I think at least I understand the mental models and the frameworks a little bit better at this point.
Hannah Clark: That's fantastic. It really sounds like you've really embraced the jobs to be done framework as a core part of that process.
Can you walk me through a little bit what that discovery process looked like and how you applied those techniques to founding Arro?
Craig Watson: So, we kind of have a process now where we start by looking at the jobs. And so when I say jobs take like research, depends on what level of detail you want to get into, but effectively you're trying to learn from your customers.
You're trying to understand what it is that they want or trying to understand what problems they have in their life, et cetera. So we'll start out by looking, okay, well, what does it actually mean in practice? What do you need to do when you need to formulate a research study? You need to decide on what it is you're trying to learn.
You then need to go out, you need to recruit, you need to think about what methodology you're going to use to engage with customers. You then need to think about how you're going to synthesize information, how you're going to take it away, learn from it, how you're going to socialize that internally, share it with the team or with stakeholders, and then how you're going to act on it.
So there's, you know, that is quite abstract. So it's quite zoomed out, but then within each of those jobs, there's a whole bunch of outcomes. So if you even think about research recruitment, what's involved there, do you need to pull a list of people from a database, understand, a customer segment?
Do you want to recruit from external panel so it's quicker but maybe it's not your existing customer base. What kind of like data compliance stuff to be conscious of engaging with customers, so it's like this laundry list of outcomes which sit underneath each shop. And it sounds a little bit dry it's almost painting by numbers in some ways but when you actually start mapping out all the steps and the different outcomes, then you can start to really have the framework to begin to understand which parts are underserved and which parts are over served.
And, you know, I've borrowed a lot from other writers and experts, people like Jobs To Be Done writers, Anthony Ulwick, et cetera, who wrote about Outcome-driven Innovation, and there's been a bunch of folks since who've improved upon that. But I think sometimes it's easy to get lost, especially in software and things like personas and maybe labels and categories, which aren't as relevant for what you're trying to learn. If you think about B2B in the space we're in, it's very workflow based, it always will be. When it comes to research, people have a job, they're going out, they're trying to fix something. And so that is how we went about it with Arro.
We mapped out the landscape, we saw where the gaps were and the thing that came back to us time and time again was there was just so much inertia when it came to customer interviews. It was really hard to get people onto a call. There was all these steps again. What were the steps in that process? First you've got to find the people, then you've got to engage with them, you've got to schedule a time, you've got to actually have the conversation, you got to transcribe the conversation, then you got to synthesize it, codify all the results, do your thematic analysis, then you've got to pay them, then you've got to do that for 50 people or whatever it is, 5, 10 people.
And then you've got to try and make sense of this aggregated lens. And it's still the most common way that research is done. And I'm a huge advocate for one-on-one customer interviews, but it just made us realize that perhaps there's a gap there. And the way that AI is moving, it's not unrealistic to think that a huge amount of those particular sub jobs related to conducting moderated interviews could be replaced or augmented by AI.
So that's really the gap that we wanted to lean into. And we've effectively built our product strategy around that.
Hannah Clark: See, so kind of building on that, what are some of those elements that you think that have a lot of potential to be disrupted by LLMs, or are you already seeing some of that disruption happening?
Craig Watson: I think the probably most obvious use cases so far, specifically in product research, have been taking on structured data and turning it into structured data. Because LLMs are just great at that, better than humans in a lot of cases, because they're effectively able to take these huge chunks of unstructured texts and across a lot of data points and turn it into something that's meaningful, accurate, and for the most part, right but not always.
But what I think teams are getting better at is things like attribution, being able to see this chain of evidence between who said what and understanding how that relates to a particular team. So a lot of the early wins, I think in the space I've been focused mostly on that kind of synthesis piece. And I think what's probably going to evolve and the reason we actually leaned a bit more into the generative side, if you think about what Arro does just for context, it's a way for people to carry out interviews, kind of AI made conversations with participants.
You set out some questions, the AI to have the conversation, and then it will take the insights, it'll synthesize those, and it will provide a report in terms of what's been learned across 50 or 100 or 1000 people. That's one advantage of AI, you can scale it much easier. So we wanted to spend a little bit more time on the generative AI piece, because the actual interviews are more generative in nature, and I think that's super interesting.
One other piece that we're starting to think about as well is like vision APIs, so taking a multimodal as well. And being able to understand how people are navigating on a UX, how they're navigating through a product. I think that's going to become much more of a feature in the next 12 to 18 months because the tech is catching up now.
And there's already, if you think again of the jobs framework, there's a ton of tools which are using things like iframes to understand how people are navigating through a Figma prototype. But it probably doesn't make sense in a couple of years to be doing it that way. It'd be much easier and cheaper and more accurate to just get an AI to watch what's happening in the User Vision API, potentially even moderating usability tests, and then come back with those insights. So two things that we're looking at.
Hannah Clark: Yeah, it's so fascinating and things that we would have not thought were possible even a couple of years ago. So that's really interesting to see it moving that quickly in that direction.
So what are some of the other ways that you see product teams using AI to their advantage in 2024 in order to be more effective during that product discovery phase?
Craig Watson: There's quite a few, I've talked to, we've probably done our own customer interviews are probably up to about 150, 200 of the stage. So we've spoken to a lot of product people, user researchers, product designers, product managers. I'm actually sometimes surprised how infrequently AI is used.
I know it sounds like a bit weird, given that in tech, most people are early adopters are quick to adopt the latest innovation, but I think there's still a little bit of a hold up for people to adopt it in the workplace. And that could be very, well, there's a variety of different reasons.
Sometimes it's internal mandates. It can be like security concerns. It can be just like, not sure of the use case. I think we're very much, even though we're in a bubble in the tech world, like we did a survey recently where it was a small enough samples, like a hundred folks, so, you know, not hugely representative, but it was interesting to get some directional learnings.
A lot of people are still unsure of how to get the most of AI in the workplace. In their personal life, they might be using ChatGPT or playing with Perplexity or they've played with Midjourney and they're exploring and experimenting. But it's still very early days. It's such early innings.
And I think that's what excites me the most is sometimes you think, Oh, this is a high everywhere. If you go on Twitter it's just like everything is AI and it feels like it's taken over the world and AGI is just around the corner, but it's so early. And actually in the B2B context, it's even earlier.
So I think that's super interesting as well. Just what could happen there? I think just to get to your question about what ways we've seen people using it. A lot of it comes to, I think it's content generation is probably the most common use case name. So it might be things like helping to write user stories if you're a product manager.
It could be helping to formulate like a research study, a proposal if you're a researcher, creating a discussion guide. It can be also, I think on the synthesis side, people have started using it, not necessarily through dedicated tools, but even just like dropping in a transcript or actually this would be a good example is like having customer conversations using a transcript from Zoom or some other automated transcript.
We're using a tool called Grain to record all of our videos and it's excellent because what it does is it'll have all of the conversation transcribed, but then you can actually layer in your own data model and ask questions of the transcripts, which is fascinating, right? You can ask like what jobs to be done and were, were identified in this conversation or can you tell me what kind of personas you think came out of this conversation?
So starting to see those two ends of it, I think either creating the content or reducing the effort in understanding the content, they're probably the most common ones. But again, it's just, it's the charity days, which is exciting.
Hannah Clark: Very exciting. And it makes you think of all the time that researchers in the past have had to really labor to synthesize a large swath of information that might be very disorganized in nature.
You're dealing with people's human responses. So it's just even just eking out some of those more nuanced insights can be really difficult to pour through, especially if you've done a lot of research for any number of products. So very interested to see how that goes as well. So when we talk a little bit about some of those same tools, I think a lot of that also applies to finding product market fit.
And I'm wondering if there is anything that you suspect is on the horizon in terms of AI assisting product teams to find fit.
Craig Watson: It's a good question. Product market fit is one of those controversial ones, having run startups for so long now. It's this holy grail that everyone aspires to get to, but it's always like a never ending journey. You never quite hit the destination, the market changes or the product changes. I think it's hard, honestly, to say that there's one particular approach or two that's going to help you get there. I think it's like a compound play where you've got different ways that you can start to move towards it.
And to give you a concrete example, I mean, there was like the famous surveys that were going around a few years ago, I think it was Rahul Vohra from Superhuman was doing like the product market fit questionnaire and it was like segmentation. And it was the jazzy way at time to go out and try and learn quickly, if you had product market fit and, there is some merit for sure to the methodology. You understand how disappointed people will be if they, if your product was taken away, but that's like a temperature check doesn't actually necessarily help you get there.
And I think probably one of the big learnings from Spotify was just how long it can take to change the inflection of like your growth curve. Like I was actually, this is public knowledge, but there was an internal podcast called The Curve for that exact reason, which Gustav Söderström, the CPO used to interview different people around the company.
And I think like I was there for five years, Gustav's been there I think 12 years or something. Now he's basically being one of the most impactful executives in the company. And I would say his takeaway over those 12 years is to get to product market fit. You've got to spend 12 years making bets, doing experiments, making the right decisions.
It's not something that's going to happen quickly. It's not like any tool can get you there. It's the intricacies of all those different pieces coming together. But what I think AI is very well placed to do is to reduce the time in all those tasks and not necessarily give you like this perfect solution that's just going to happen quickly, but it's going to speed up the time to getting some of those tossed on, if that makes sense.
Hannah Clark: It makes sense. Yeah. And just calling back to that whole idea of falsification. And I agree, I think that's a huge challenge that a lot of folks have a hard time kind of owning their egos and putting that at the door. But I want to talk a little bit about the suitability of AI features in some products.
I think that right now there's a little bit of shiny object syndrome situation happening with a number of different companies that are established or startups as well. There seems to be a lot of pressure to integrate AI features into products because it seems to be this table stakes situation. But I'd argue that I've seen some executions of AI integrations that just, in my opinion, haven't added a lot of value.
And I think that that we see that more and more these days. So how might one go about understanding, you know, what, whether it's necessary even to use AI or whether it's even worth the investment of adding an AI feature to a product that exists and assessing whether there's value in that investment?
Craig Watson: Yeah, I think we've all seen a few pretty scary use cases where, you know, chatbot has tried to sell you a car or go off the rails and it's not nice when it happens and it goes public pretty quickly and it's, there's a huge brand impact for companies if they get that wrong. So, I mean, I think people are right to question the use cases and also to make sure it's done in a compliant way and like an ethical way.
I think the way that I've seen most companies try to force it in, again, as it's probably gets back to what we talked about is when you're in solution mode and you don't really understand what the customer is trying to get us with the particular job in your software. So take an example, if you are building like a, I'll take a like Notion as an example. We use Notion as our kind of as our knowledge base across where remote company.
So we rely on it quite a lot, share a lot of written documents. I think that's a, obviously a very great fit for AI, because there's times when I just want to write an outline and I can ask it to continue writing or to improve my writing. And it like really is right into my workflow. There's a quick feedback loop there.
I can fix it and I can move on. I think where you like, you suggested shiny objects syndrome kicks in as people just not really understanding what the end user is trying to achieve. And if you're optimizing something that doesn't really help them in their job anyway, it just looks kind of cool or it's interesting.
It's never going to really improve things. It's not really going to help, right? So I guess for what we have learned is again, is just always getting back the first principles of understanding what someone trying to achieve here and how would AI either speed up the flow or reduce the cost or make some other kind of efficiency that was worth the investment from the product development side.
And it's not a guarantee, you really do have to sense check. Is this worth investing in? Sometimes the small delightful pieces are worth investing in. And like we have personally spent months trying to get the conversational UX of Arro right, because that's the core engine of our product. It's like going out and talking to people.
And it's right that we spent 80%, 90% of the time on that. And we haven't spent the rest of the time, trying to include random little features of AI across the product. So it looks cool and you can flick the little wand and the magic button and something nice happens. It's like, yeah. So I think it just, it always comes back to what are people trying to achieve? How can you speed that up? Or how can you improve it? And if AI is a good fit for that, then absolutely it's worth the investment.
Hannah Clark: I would agree wholeheartedly. So on the topic of making some maybe a little sketchy decisions, whether with investing time or money, what are some of the other caveats as a founder yourself that you would warn other founders or product managers to be mindful of during the discovery phase and particularly in today's landscape?
Craig Watson: I think it's quite situational, like it's context dependent on what stage you're at. Like if you're really early stage, it's very much almost trying to find the right market that you can insert your product into and understanding that market really well. Sometimes people are building a startup on a problem they have.
So they know the problem, but other times they like a particular market and they want to attack it and find some interesting ways to go about it. The worst way is you build some solution and you then try and find like a problem that it will fit to, but that does happen as well. So I think depending on the stage you're at, you know, it's different if you're a scaling company, like a lot of the customers who are using Arro now tend to be scale ups and their problem is that they have a mature product or, but they don't necessarily have the research bandwidth to go out and understand how they might build a new feature.
So uses something like R2 helps them scale up their research efforts and get in front of more customers so they can be able to bring in more insights. But arguably, I would say I was not a great fit if you're like a pretty early stage because I actually think relationship building is so important, getting in front of potential customers, learning about their pain points and doing that generative research that's around your value side is where you need to be.
So I guess it's a little bit dependent on each case, but I think that would be my advice is, it's not one-size-fits-all. And there's some great books out there. Teresa Torres has written about Continuous Product Discovery. Michele Hansen wrote a book around how to talk to customers. Some of the Jobs to Be Done stuff is great.
It really depends on the stage you're at, but there's so many great sources out there, even compared to when even five years ago, I think because the product discovery has come a huge way in terms of know how. So, yeah, there's something out there for everybody.
Hannah Clark: Yeah, I would agree. We just had a great episode with Steve Portigal who wrote interviewing users and really interesting insights about even just the psychology of speaking to people and being able to get the best insights without inserting yourself into the conversation and affecting the outcome.
So yeah, this has been a great conversation, Craig, I really appreciate you coming to join us today. Where can listeners follow your work online?
Craig Watson: So you're welcome to check out Arro itself, it's www.arro.co. Also, feel free to connect with me on LinkedIn, it's just Craig Watson. I'm one of the co-founders of Arro, or on Twitter, it's cdcwatson is my handle.
So I'd love to chat with people, any questions, happy to connect.
Hannah Clark: Well, thank you so much for coming on. We really appreciate it. And thank you so much for sharing all your insights.
Craig Watson: It was a pleasure. Thanks for having me, Hannah. Great talking with you.
Hannah Clark: Thanks for listening in. For more great insights, how-to guides and tool reviews, subscribe to our newsletter at theproductmanager.com/subscribe. You can hear more conversations like this by subscribing to the Product Manager wherever you get your podcasts.